Lossless Convexification
Many autonomous guidance problems require us to find a path to a goal, while ensuring that collisions do not occur, and minimizing a cost functional such as fuel. One example of such a problem is the powered descent guidance problem for Mars pinpoint landing. While such guidance problems are naturally posed as optimization problems, the use of optimizers in autonomous spacecraft has been limited by the fact that general nonconvex optimization approaches do not guarantee finding a solution of one exists, converge only to local minima and are sensitive to initial guesses.
Convex optimization is a promising tool for onboard autonomy, because it provides guaranteed convergence to the globally optimal solution. However many problems, such as the Mars pinpoint landing problem, do not have the convex structure required to employ convex optimization. In particular, the Mars pinpoint landing problem has nonconvex control constraints due to there being a lower bound on the achievable spacecraft thrust. My research with Behcet Acikmese has overcome this through a technique called "lossless convexification". The key idea is to reformulate the nonconvex problem as a higher-dimensional convex problem, and then prove that an optimal solution to the convex problem is also a globally optimal solution to the nonconvex problem. We refer to this as "lossless" because no region of the feasible space is removed in the convexification. In other words, if a feasible solution to the nonconvex problem exists, we are guaranteed to find it.
Lossless convexification has been implemented as the 'G-FOLD' (Guidance For Optimal Large Diverts) algorithm, and demonstrated on the Masten Aerospace Xombie rocket. This experiment showed that, via lossless convexification, convex optimization can be used to solve precision landing problems on board and in real time.
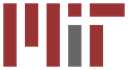
Related Publications
"Lossless Convexification of Non-Convex Control Bound and Pointing Constraints of the Soft Landing Optimal Control Problem ." B. Acikmese, J. M. Carson III, and L. Blackmore. IEEE Transactions on Control Systems Technology, Volume 21, Issue 6 (2013).
"Lossless Convexification of Control Constraints for a Class of Nonlinear Optimal Control Problems." L. Blackmore, B. Acikmese and J. M. Carson III. Systems and Control Letters 61 (2012), pp. 863-870.
"Lossless Convexification of Control Constraints for a Class of Nonlinear Optimal Control Problems." L. Blackmore, B. Acikmese and J. M. Carson III. In the Proceedings of the 2012 American Control Conference.
"Lossless Convexification of a Class of Optimal Control Problems with Non-Convex Control Constraints." B. Acikmese and L. Blackmore. Automatica Vol. 47, No. 2, Feb 2011, pages 341-347.